Neural network verifies proton dose directly from PET detector data
20 Oct 2022
Accurate predictions Top row (left to right): dose distribution for a 110 MeV proton beam generated using the AI model; the true dose distribution; and the absolute difference between the two. Bottom row (left to right): positron profile generated using the AI model; the true simulated profile; and the absolute difference. (Courtesy: CC BY 4.0/Phys. Med. Biol. 10.1088/1361-6560/ac8af5)
To maximize the quality of proton therapy delivery, researchers are generating precise, rapid and optimized methods for in vivo dose monitoring and range verification in proton treatments. This is no easy feat.
During proton therapy, high-energy protons in the treatment beam interact with nuclei in the patient to produce prompt gammas and short-lived positron-emitting isotopes, which emit pairs of 511 keV gammas that can be detected using positron emission tomography (PET). The trail of isotopes left in the wake of the proton beam follows the dose distribution closely. When the proton energy drops below a threshold for an isotope’s production, the trail stops.
Methods to obtain accurate positron emission activity distributions must consider photon attenuation, PET detector efficiency, the uncertainty introduced by nonlinearities in positron range, and randomness and scattering within a detector. And after researchers reconstruct the positron emission activity, they need to map the activity profiles to dose distributions, another complicated process.
To date, methods for verifying proton dose from PET detector data fall into one of two classes. Indirect methods detect the secondary radiation emitted from the patient when charged particles interact with tissue and compare the measured and precalculated distributions of these secondary particles. These methods do not directly estimate the difference between delivered and planned doses, however, and therefore aren’t ideal for evaluating treatment progress.
Direct methods provide dose and range verification throughout a patient using the activity profiles of the positron emitter. Unlike indirect methods, direct approaches don’t require traditional reconstruction schemes. Researchers have also investigated statistical approaches that can be performed directly or indirectly.
Recently, researchers at National Taiwan University, National Central University and the Institute of Physics at Academia Sinica applied deep-learning methods to directly map PET detector data to intrinsic dose distributions in proton therapy without reconstructing the PET activity distribution. Their method bypasses traditional PET image reconstruction and demonstrates the feasibility of directly mapping proton dose from coincidence events (detection of the two 511 keV annihilation photons) using just the raw data.
The neural network method
“Understanding the dose distribution is an essential aspect of achieving high treatment quality in particle therapy,” says Mythra Varun Nemallapudi, second author on the Physics in Medicine & Biology study. “Currently, there aren’t many verification systems installed in facilities around the world, as this is still in the R&D phase…The key to our solution is to train neural networks to learn both the secondary particle distribution of 511 keV photons emitted from positron annihilation isotopes created from proton interactions [with tissue], and the dose distribution with the aid of simulations.”
Nemallapudi and his team used Monte Carlo simulations to generate datasets of an abdominal phantom irradiated with high-energy protons and imaged with compact in-beam PET detectors. They then employed a neural network model based on conditional generative adversarial networks (cGANs) to provide the most likely dose distribution given the PET activity distribution at the detectors.
Proton simulations Left: simulation setup for irradiation with a mono-energetic beam (centre) and a spread-out Bragg peak (lower centre). Right: the detector configuration. (Courtesy: CC BY 4.0/Phys. Med. Biol. 10.1088/1361-6560/ac8af5)
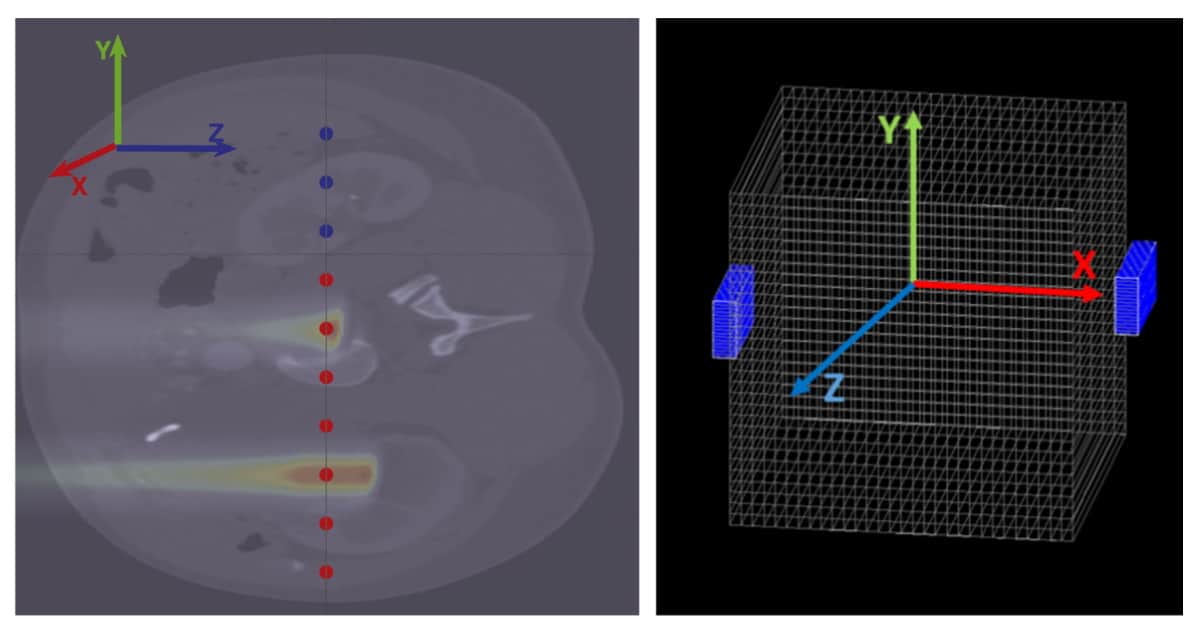
The neural network was trained for underlying imaging physics, detector geometry and detector non-uniformity directly from experimental data. The model was also trained on a diverse set of data containing different energies, positions and spot sizes to help reduce the chance of model divergence, a common challenge with cGANs. The researchers didn’t apply the model to simulated tissues with large inhomogeneities, such as the lungs, as that would increase model output uncertainties.
The researchers assessed the model’s performance using several approaches, including an evaluation of the shift in Bragg peak position (the depth at which the proton beam deposits most of its dose) for monoenergetic irradiations between 50 and 122 MeV. Results were obtained using 10,000 coincidences acquired five minutes after proton irradiation. They found that the trained model could effectively map the proton dose from detected coincidences – the model output deviated from true dose and range values by 1% and 2%, respectively.
The trained model performed well despite the low number of counts produced in compact detectors. This level of performance is critical because traditional PET systems are too bulky for installation in current proton therapy facilities. Compact detectors, on the other hand, can be integrated with the proton treatment gantry and positioned close to the patient.READ MORE

The researchers now plan to implement their model on treatments using multiple fields at different angles. Such setups will augment current challenges related to the limited number of coincidences available for mapping PET activity and complex dose distributions.
“Imaging secondary gammas is very promising; however, there is a need for practically adoptable detector systems,” Nemallapudi says. “In addition to dose, there is a plethora of information pertaining to the treatment waiting to be unleashed from the patient. I believe that researchers, clinicians and the public will all profit from continuing this line of research using practical systems that expand on functionality.”
He notes that higher proton fluxes and a higher number of coincidences can be expected as researchers investigate hypofractionated therapies and FLASH for proton therapy.
Catherine Steffel is a science writer based in Madison, Wisconsin. Catherine was previously a PhD student contributor to Physics World.
FROM PHYSICSWORLD.COM 20/10/2022
Δεν υπάρχουν σχόλια:
Δημοσίευση σχολίου