Computational model maps patterns of neurodegeneration across the brain
12 Apr 2022 Simon Lichtinger
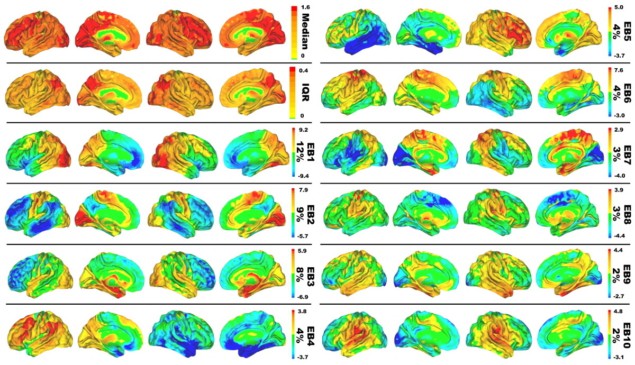
Memory loss, difficulty finding words, trouble with navigating even in familiar neighbourhoods. These are just a few of the dementia symptoms experienced by an estimated 50 million people worldwide. Of those patients, whose number is projected to triple by 2050, two thirds suffer from Alzheimer’s disease. This neurodegenerative disorder is caused by accumulation and aggregation of amyloid beta and phosphorylated tau proteins in the brain. But how the resulting toxicity to brain cells gives rise to the complex and diverse clinical symptoms of Alzheimer’s is only just beginning to be understood.
Researchers at the Mayo Clinic in Rochester, MN, have now developed a computational model that maps large-scale patterns in brain metabolic activity to clinical data. Their results, published in Nature Communications, indicate that including just a few global correlation features is sufficient to derive an accurate model of clinical status from a three-dimensional image of brain activity.
The researchers used brain scans of over 400 Mayo Clinic patients with Alzheimer’s disease as the backbone of their computational investigation. In preparation for the scans, clinicians inject patients with the radiotracer 18F-fluorodeoxyglucose (FDG), which accumulates in metabolically active cells. They can then employ positron emission tomography (PET) to obtain a three-dimensional map of cellular energy usage. This technique (known as FDG-PET), is commonly applied to image cancers, but also lights up glucose-hungry brain cells.
“FDG-PET is sensitive to neurodegenerative diseases of mental function at the single subject level and is currently used in clinical practice,” explains lead author David Jones. “[This] likely relates to a fundamental relationship between brain function and energy metabolism that reveals the functional neuroanatomy relevant for dementia symptoms.”
FDG-PET scans generate a very high-dimensional data space. Each image voxel carries information that can distinguish different patients from each other, and extracting useful knowledge requires a dimensionality reduction technique. In this work, the scientists employed principle component analysis (PCA), a mathematical tool that can identify the patterns of variation in high-dimensional datasets that explain most of the statistical variance. By applying PCA, they were able to represent 51% of the inter-patient variance (in glucose uptake across the brain) in just 10 patterns, which they called “eigenbrains”.
Because any brain scan can be represented within this reduced coordinate system (as a unique combination of the 10 brain glucose patterns), the authors next constructed a linear model to correlate the scans to patient information, such as age or clinical dementia rating. In unrelated validation datasets, the model could infer a patient’s age just using the FDG-PET image, with a mean error of five years. The model could also predict the presence or absence of clinical dementia with an accuracy of over 90%, even though it was never specifically optimized for such tasks.
An important consideration in the choice of mathematical model is interpretability, that is whether or not one can understand the model’s behaviour at all stages. As Jones emphasizes: “For medical decision making, it is critical to have an interpretable model. In some sense, every patient encounter has some ‘edge case’ features present. Having an interpretable model allows for medical reasoning within the context of the patient that is in front of you, at the time you are making medical decisions.”
While the researchers did not perform a rigorous interpretation of the eigenbrains in the study at this stage, they hypothesized that the most important eigenbrains might correspond to whether the brain is occupied with internal versus external, or abstract versus concrete, information. As a consequence, the model captures general mechanisms of information processing in the brain. By viewing conditions such as Alzheimer’s not merely as a protein folding disease, but as a spectrum of effects on wider brain functions, models like this one can also inform scientists about the workings of the healthy brain.READ MORE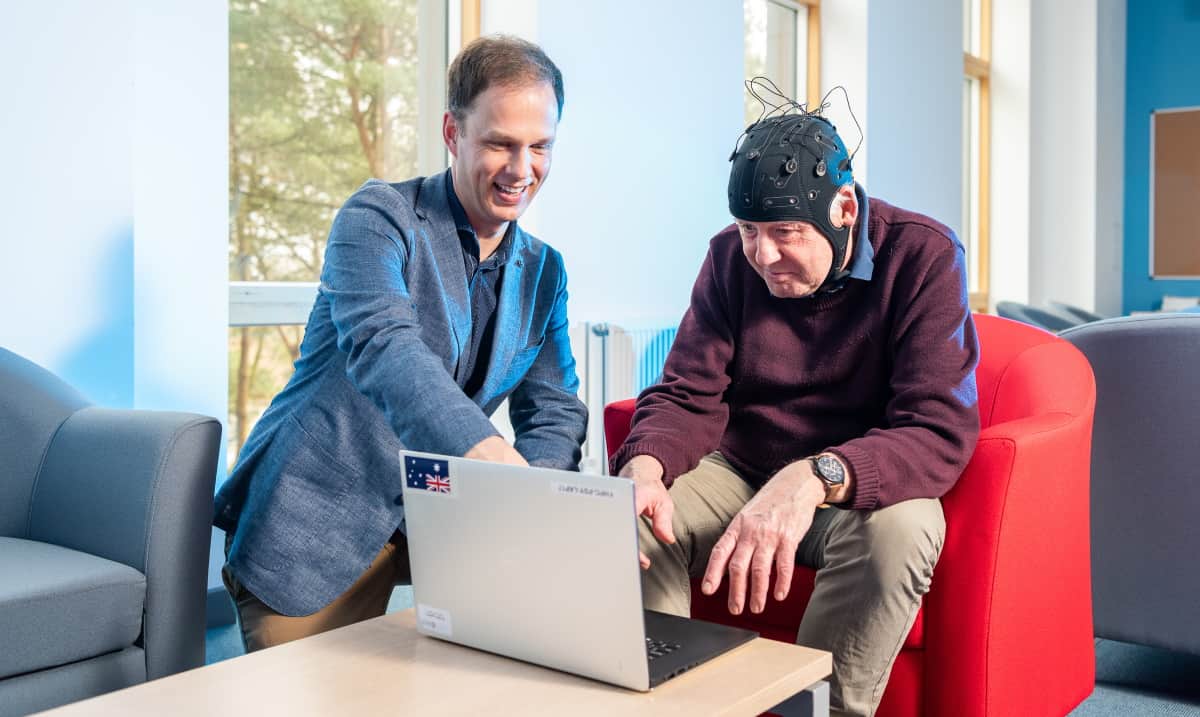
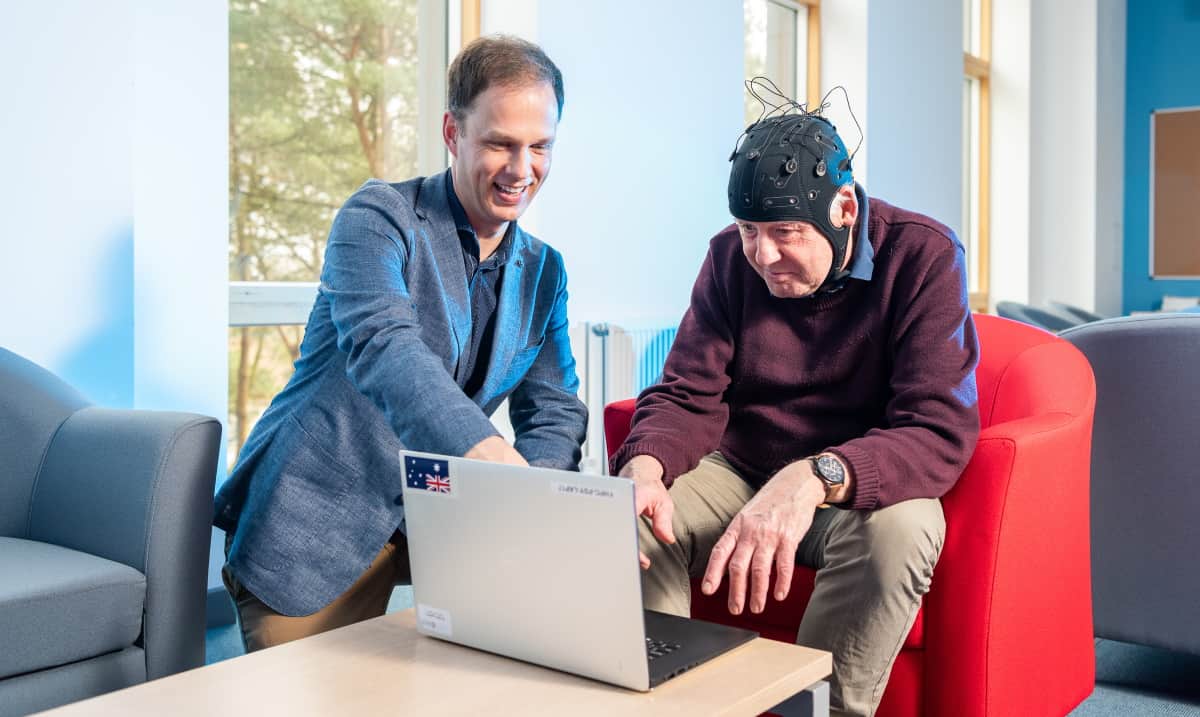
“This model can inform new taxonomies of degenerative dementias and the mind in general,” Jones concludes. “In addition to making the interpretation of brain scans from patients easier by only needing to look at a few anatomic patterns rather than hundreds, this new model may advance our understanding of how the brain works and breaks down during aging and Alzheimer’s disease, providing new ways to monitor, prevent and treat disorders of the mind.”

Simon Lichtinger is a PhD student contributor to Physics World. Simon is at the University of Oxford, where he uses molecular simulations to study drug transport through membrane transporter proteins. Find out more about our student contributor networks
from physicsworld.cpom 15/4/2022
Δεν υπάρχουν σχόλια:
Δημοσίευση σχολίου